A brief introduction to AlphaFold
A beginner's introduction to AlphaFold, the AI from DeepMind that predicts protein structure. The first article in a longer series about bioinformatics.
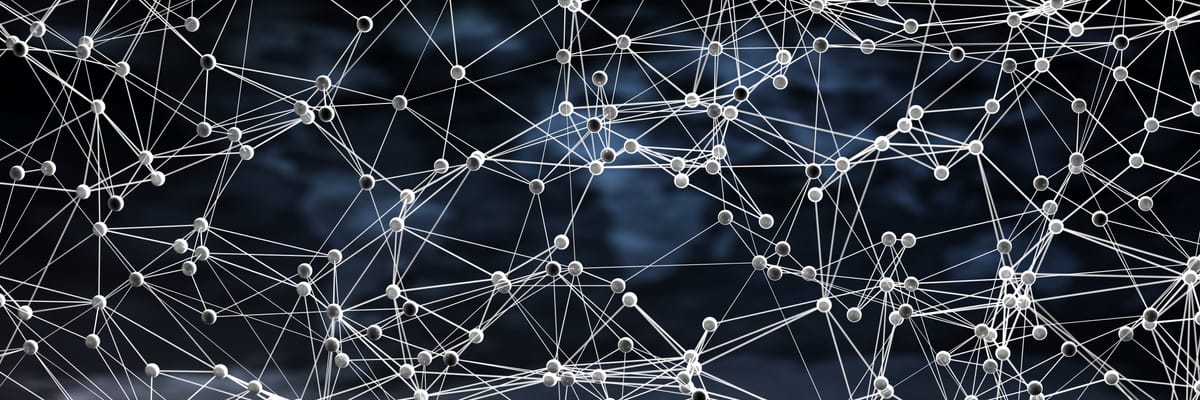
In July earlier this year, the code, methodology, and database behind AlphaFold, the protein prediction AI software developed by DeepMind, was made open source through the publication of two articles in Nature.
AlphaFold is a major advancement in the quest to predict a protein’s structure from its sequence alone. In nature, proteins reliably fold into precise 3D conformations that is critical for its function based on nothing more than the sequence of amino acids that it is composed of. In fact, mutations in proteins that lead to misfolding are often associated with disease states, for example, Alzheimer’s and Parkinson’s. However, we have not been able to understand this folding process nor predict the 3D shape of a protein based on its sequence alone.
Although we have currently found sequences for millions of proteins, we have only solved the structures of about 180,000 of them. Structural biology techniques have been developed to solve structures experimentally: x-ray crystallography, nuclear magnetic resonance, and cryo-electron microscopy. These methods involve large amounts of trial and error and have been limited in the complexity of proteins they can be applied to. Outside the lab, computational methods have been developed to predict how a protein may fold based on its sequence to bypass the experimental resources. However, these traditionally relied on using templates from experimentally-solved structures, which then imposes the same limits on the range of proteins they work best for.
AlphaFold 2, which uses deep learning algorithms to predict structure to atomic accuracy (within 1 Å or 0.1 nm of error), has been the most successful computational approach so far. In brief, AlphaFold operates with three main parts. The first involves constructing an initial model for which amino acids may be in contact with each other in the folded protein. Second, it uses a machine learning method called attention to interpret which parts of the model are informative, it takes the informative parts of the model to reconstruct an improved model for amino acid contacts, and the improved model is reinterpreted. This process occurs iteratively for a number of cycles, then the final improved model is fed through the third part which produces the 3D model of the protein. The software will feed the predicted 3D structure back into the second step, and this loop occurs several times for the model to be refined.
The final output of AlphaFold is a file containing the 3D coordinates for every non-hydrogen atom in the protein. It also outputs a graph showing the confidence levels for every amino acid residue, which allows users to assess the reliability of the predicted structure.
AlphaFold is an outstanding contribution to the field of bioinformatics. In the most recent blind assessment of structure prediction software (the CASP14 initiative), it significantly outperformed competing approaches. It is considered to be the closest we’ve gotten to solving the structure prediction problem.