Hopfield and Hinton awarded Nobel Prize for shaping the neural network landscape
John Hopfield and Geoffrey Hinton are recognised for their seminal work in artificial neural networks and machine learning.
The Nobel Prize for Physics in 2024 has been awarded jointly to Professors Emeriti John Hopfield, of Princeton University, and Geoffery Hinton, of the University of Toronto, “for foundational discoveries and inventions that enable machine learning with artificial neural networks”.
The physics laureates used physics to find patterns in information. Using tools from physics, they constructed methods that laid the foundation for machine learning.
John Hopfield, well known for developing the “Hopfield Network”, created a structure that can store and reconstruct information.
Geoffery Hinton, also known as “the Godfather of AI”, invented a method that can independently discover properties in data, which has become an important feature in large artificial neural networks (ANNs).
Their work has demonstrated a new way for us to use computers in order to tackle scientific problems.
ANNs and machine learning date back to the 1940s, when Warren McCulloch, Walter Pitts and Donald Hebb proposed mechanisms describing how neurons in the brain cooperate. Only within the past three decades, these methods have been developed into versatile and powerful tools for scientific applications. Inspired by neurons in the brain, ANNs are large collections of nodes, analogous to neurons, each connected by weighted couplings, analogous to “synapses” which are trained to perform certain tasks – rather than carrying out a set of predetermined ones. The structure of ANNs is closely related to spin models seen in statistical physics. This year’s laureates exploited this connection to make breakthrough advances in ANNs.
Hopfield’s seminal work in biological physics in the 1970s examined electron transfer between biomolecules and error correction in biochemical reactions. In 1982, he published a paper describing a dynamic, associative memory model based on a simple neural network, called the Hopfield network. This is a network that can store patterns and is capable of recreating them. Hopfield likened his network that searches for patterns to a ball rolling down a landscape of peaks and valleys. If a ball is dropped into the landscape, it will roll into a valley. For a network, it is given a pattern, analogous to a ball rolling down a hill, into a valley – and will search for the nearest pattern stored in its memory, analogous to a ball ending up at the bottom of the valley in the energy landscape. When the network is trained, it creates a valley in a virtual energy landscape for every saved pattern.
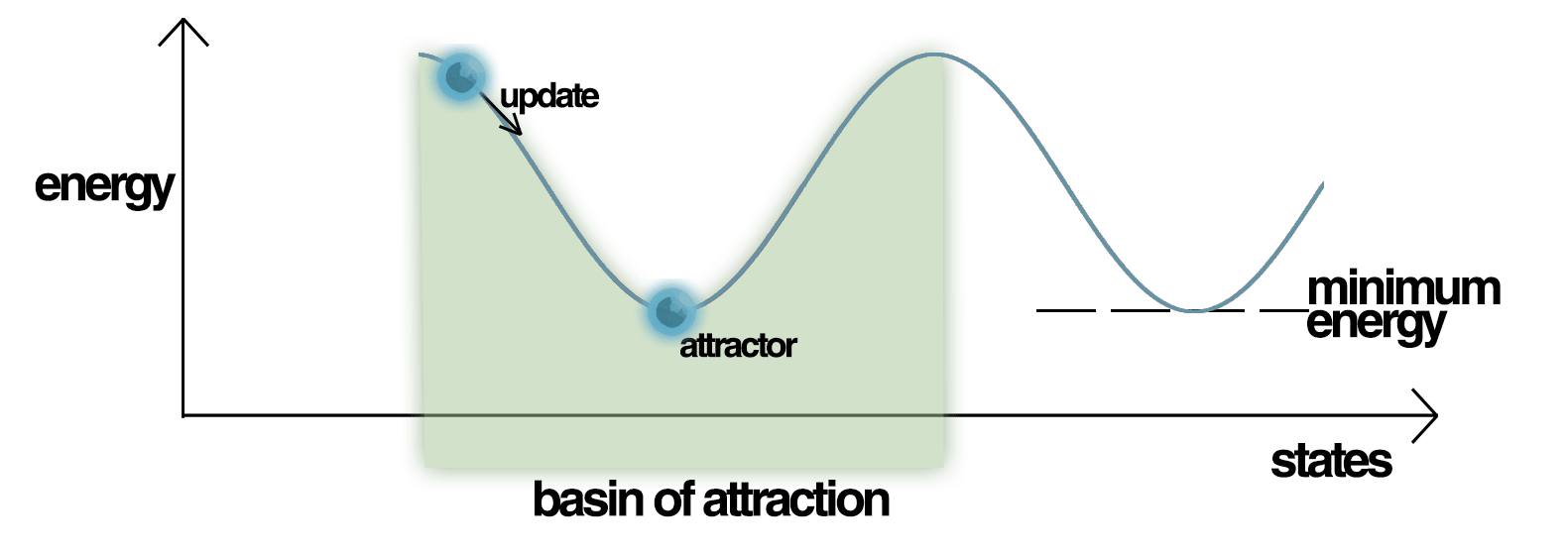
In 1983-1985, Geoffrey Hinton developed a stochastic extension of Hopfield’s model, called the Boltzmann machine. This is a generative module, which focuses on statistical distributions of patterns rather than individual patterns.
These pioneering methods developed by the laureates have been instrumental in shaping the field of ANNs. Their work has demonstrated a completely new way for us to use computers in order to tackle scientific problems. ANNs now have become a standard data analysis tool used in astrophysics and astronomy, they were use for the search of the Higgs boson is CERN, and most secularly - deep learning ANNs methods are used as the AlphaFold tool for prediction of three-dimensional protein structures, given their amino acid sequences.
The laureates will pick up their awards at the Nobel award ceremony later this year on the 10th December.