Classroom to Curbside: Insights from Harvard’s Food Truck Challenge
An Imperial Business student’s experience and review on business strategy and analytics
The Food Truck Challenge by Harvard Business School is a simulation which has students play the role of a food truck entrepreneur setting up and managing in a competitive ever-changing environment. Through my experience in the exercise, I uncovered two key insights that extend beyond the simulation itself and into the domain of practical challenges businesses face. I then looked at the relevant business literature to compare.
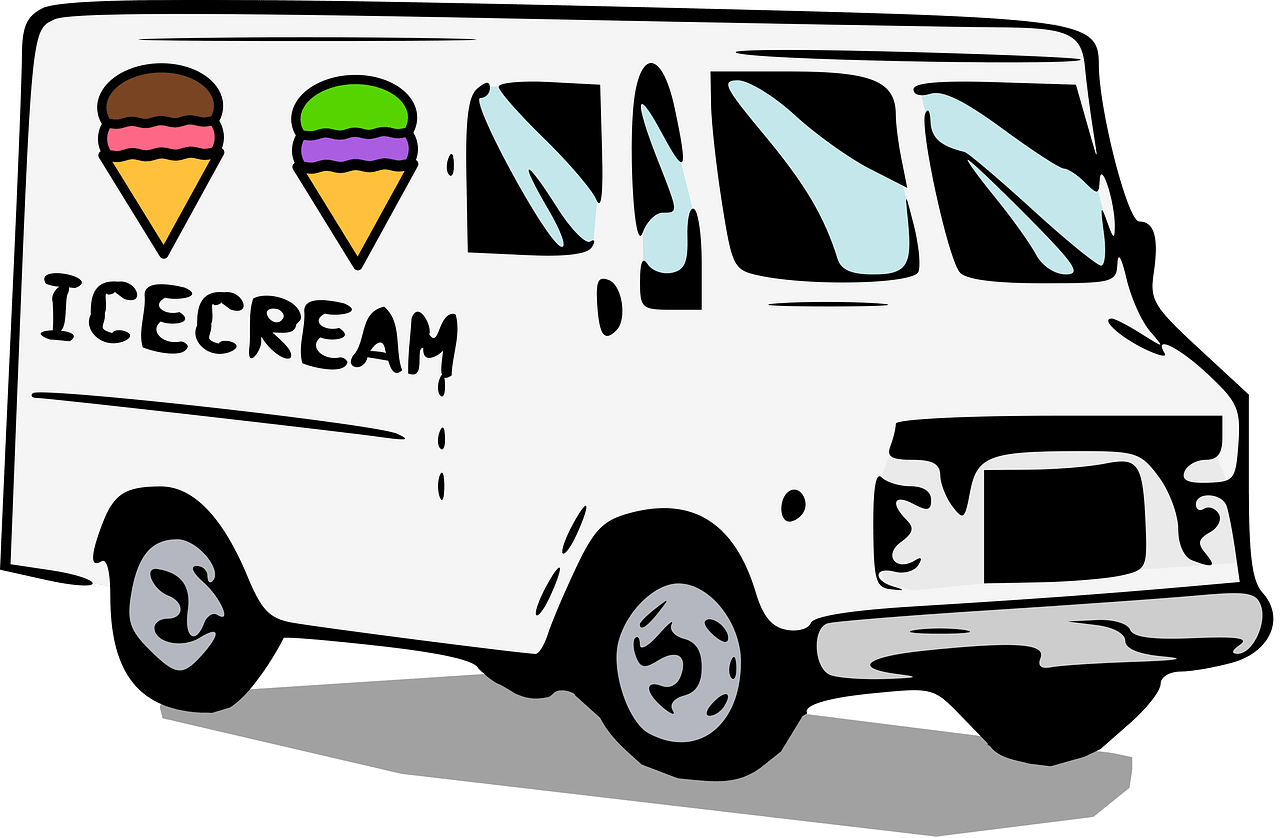
Key Insight: The best business plans collide with reality - make one still
Situation: The utility of a business plan was tested during the simulation. Initially, my team began by strategising, analysing all the available data, and devising a plan that would position us in the optimal location with the ideal food selection. We decided to place our truck near the college campus, serving ice cream. Confident in our choice, backed by the data we submitted our selection—only to discover that construction on campus significantly reduced foot traffic, which led to lower sales. All our carefully laid-out business plans were rendered ineffective.
Literature: If, in the immortal words of Steve Blank, famed entrepreneur and progenitor of the Lean Startup methodology, “No business plan survives first contact with a customer,” why does the practice persist? A more expansive view suggests that the concept of a business plan as a purely strategic document is uncorrelated with success. However, as a planning exercise that forces entrepreneurs to think through the process of launching a new business, research validates its utility.
Business plans create a mental model for entrepreneurs and can help mitigate cognitive biases. Although the business plan is almost certainly subject to change, by creating this mental model, it allows entrepreneurs greater mental flexibility to adapt to changes. Interestingly, other traditional business planning activities, such as “gathering secondary data or sharing the business plan for feedback or funding,” are not correlated with success. As an aside, the research validates my team’s decision not to pursue the research gathering option in the simulation and instead jump into prototyping by using the cart in the first week. This allowed us to iteratively develop our business strategy and exemplifies the Lean Startup methodology.
Key Insight: Possess a healthy skepticism of analytics
Situation: When first tasked with the simulation, my teammates and I referred to taste preferences published in the provided market data. However, we quickly discovered the data didn’t seem to be entirely accurate. Contrary to the report, young adults weren’t prioritising healthier options, and seniors seemed just as enthusiastic about ice cream as the children. Quickly, we recalibrated our use of the market data, deciding its outdatedness and possibly idealised self-reported insights were far less relevant than the immediate data on revealed preferences.
Literature: It is taken for granted that analytics are valuable to any modern venture, yet according to Gartner, over 85 percent of big data analytics projects fail. Additionally, according to a McKinsey survey, only eight percent of respondent organisations were able to successfully scale their analytics beyond a specific use case. What, then, accounts for the constant pursuit of data if these projects so often fail?
In their seminal paper on big data analytics, McAfee and Brynjolfsson compared big data to traditional analytics. They find that firms that describe themselves as data-driven are, on average, “5% more productive and 6% more profitable than their competitors”. Additionally, another strength of big data analytics is its real time capabilities from diverse sources. Thus, it is clear that analytics offer firms a competitive advantage, but how and when to trust them remains a critically understudied focal point.
According to the framework laid out by Andriole and Barsky the leading causes of failure in analytics within firms are data issues, modelling issues, tools issues, talent issues, management issues, and cultural issues. Specifically, among data, modelling, and tools problems, one of the most challenging issues is obtaining timely and accurate data. For example, in the simulation, my team faced the issue that while the data might have been accurate at a specific point in time, its validity for making predictions was questionable due to changing market conditions which rendered past trends less reliable for current decision making. While analytics are rightly feted as a competitive advantage that firms can utilise, attention needs to be paid to the factors that render them inaccurate. Both theories support the original insight, that in our case, no data might have been better than inaccurate data.
The two key insights are all fundamentally related to one another in that they stress the value of context. While a business plan may collide with reality, the literature shows there are important contexts where it can be utilised strategically and analytic data can be extremely helpful, but in the wrong context, it can be maladaptive. For a venture to succeed, entrepreneurs should be encouraged to break with strict business theory and embrace whatever the context may be.
Special thanks to Professor Dmitry Sharapov for his engaging class in Innovation & Entrepreneurship and for his valuable support on the simulation, which served as the foundation for this article.