Understanding the shape of life
The 2024 Nobel Prize in chemistry was awarded to David Baker, Demis Hassabis, and John Jumper for their protein structure breakthroughs.
Proteins – macromolecules essential to life at a molecular level – are chains comprising 21 different amino acids. Without them, life could not exist. Their complexity is unfathomable, primarily because the amino acid chain, known as the ‘primary structure’, can engage in various molecular interactions within the protein. These include interactions within the chain itself (‘secondary structure’) and between unique side chains branching from different amino acids (‘tertiary structure’), causing each protein to fold into unique shapes.
With the average protein length in a human cell being 430 amino acids – and many exceeding this – understanding how proteins fold and interact to form shapes critical to their function has challenged scientists for decades, a puzzle termed the ‘protein folding problem’.
Solving this problem has profound implications for medicine and drug discovery. Many diseases result from misfolded proteins, and nearly all modern drugs target proteins in some form. Understanding protein structures is thus crucial for understanding and treating diseases.
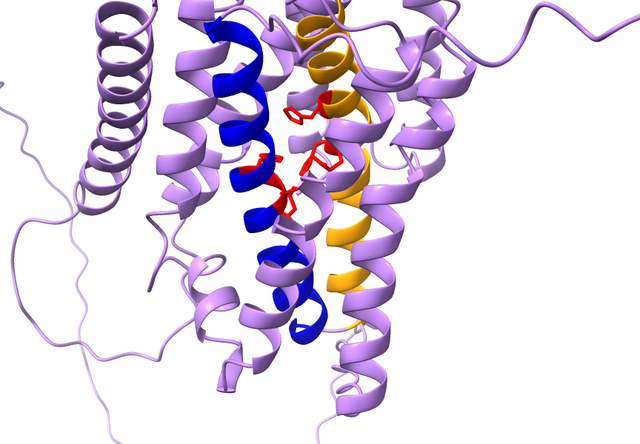
Historically, protein structures were determined using techniques like X-ray crystallography, NMR spectroscopy, and mass spectrometry. This required producing, isolating, and testing proteins, with a sanguine hope that the data would be sufficient for structure decoding. While effective for many proteins, this method failed to solve morewcomplex proteins for decades. It is also time-consuming and expensive due to the practical advanced techniques required.
But one must ask: is this a testament to AI and machine learning’s prowess or to the hands that guided it?
However, with advances in machine learning and modern technology, scientists thought it might be possible to predict protein structures computationally. David Baker’s lab believed protein structures could be predicted from amino acid sequences. Their work led to the release of Rosetta in the 1990s, which was updated significantly in 2003, allowing scientists to predict protein structures computationally. This also opened the door to designing ideal protein structures for specific tasks and reverse-engineering the amino acid sequences needed to create them. This led to engineered proteins not seen in nature, such as those inhibiting the COVID-19 spike protein and enzymes that degrade plastics.
Demis Hassabis, John Jumper, and their teams at DeepMind aimed to improve upon this using modern machine learning. They created a model trained on a massive dataset of experimentally determined protein structures. Their work culminated in AlphaFold 2’s release in 2020, a significant advancement capable of predicting virtually all known proteins with near-flawless accuracy.
The efforts of David Baker, Demis Hassabis, and John Jumper have effectively solved the protein folding problem; what once took years in the lab can now be determined computationally in hours. The impact is already substantial, with Rosetta used to design proteins for the COVID-19 vaccine and AlphaFold 2 decoding proteins in bacteria involved in antibiotic resistance.
Only time will reveal the full scope of this Nobel Prize-winning achievement. But one must ask: is this a testament to AI and machine learning’s prowess or to the hands that guided it?